The Future Fabric of Data Analysis
When subatomic particles smash together at the Large Hadron Collider in Switzerland, they create showers of new particles whose signatures are recorded by four detectors. The LHC captures 5 trillion bits of data — more information than all of the world’s libraries combined — every second. After the judicious application of filtering algorithms, more than 99 percent of those data are discarded, but the four experiments still produce a whopping 25 petabytes (25×1015bytes) of data per year that must be stored and analyzed. That is a scale far beyond the computing resources of any single facility, so the LHC scientists rely on a vast computing grid of 160 data centers around the world, a distributed network that is capable of transferring as much as 10 gigabytes per second at peak performance.
The LHC’s approach to its big data problem reflects just how dramatically the nature of computing has changed over the last decade. Since Intel co-founder Gordon E. Moore first defined it in 1965, the so-called Moore’s law — which predicts that the number of transistors on integrated circuits will double every two years — has dominated the computer industry. While that growth rate has proved remarkably resilient, for now, at least, “Moore’s law has basically crapped out; the transistors have gotten as small as people know how to make them economically with existing technologies,” said Scott Aaronson, a theoretical computer scientist at the Massachusetts Institute of Technology.
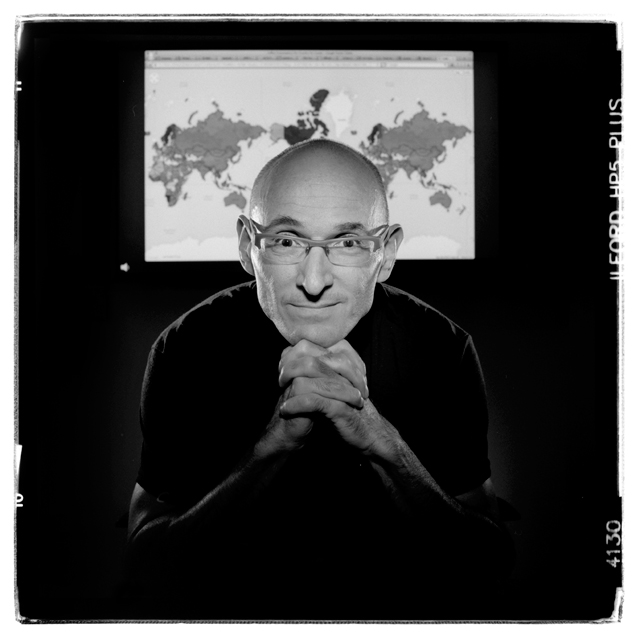
Instead, since 2005, many of the gains in computing power have come from adding more parallelism via multiple cores, with multiple levels of memory. The preferred architecture no longer features a single central processing unit (CPU) augmented with random access memory (RAM) and a hard drive for long-term storage. Even the big, centralized parallel supercomputers that dominated the 1980s and 1990s are giving way to distributed data centers and cloud computing, often networked across many organizations and vast geographical distances.
These days, “People talk about a computing fabric,” said Stanford University electrical engineerStephen Boyd. These changes in computer architecture translate into the need for a different computational approach when it comes to handling big data, which is not only grander in scope than the large data sets of yore but also intrinsically different from them.
The demand for ever-faster processors, while important, isn’t the primary focus anymore. “Processing speed has been completely irrelevant for five years,” Boyd said. “The challenge is not how to solve problems with a single, ultra-fast processor, but how to solve them with 100,000 slower processors.” Aaronson points out that many problems in big data can’t be adequately addressed by simply adding more parallel processing. These problems are “more sequential, where each step depends on the outcome of the preceding step,” he said. “Sometimes, you can split up the work among a bunch of processors, but other times, that’s harder to do.” And often the software isn’t written to take full advantage of the extra processors. “If you hire 20 people to do something, will it happen 20 times faster?” Aaronson said. “Usually not.”
Researchers also face challenges in integrating very differently structured data sets, as well as the difficulty of moving large amounts of data efficiently through a highly distributed network.
Those issues will become more pronounced as the size and complexity of data sets continue to grow faster than computing resources, according to California Institute of Technology physicist Harvey Newman, whose team developed the LHC’s grid of data centers and trans-Atlantic network. He estimates that if current trends hold, the computational needs of big data analysis will place considerable strain on the computing fabric. “It requires us to think about a different kind of system,” he said.